Casual Info About How Do You Find The Linearity Of A Line Excel Chart Move Axis To Right
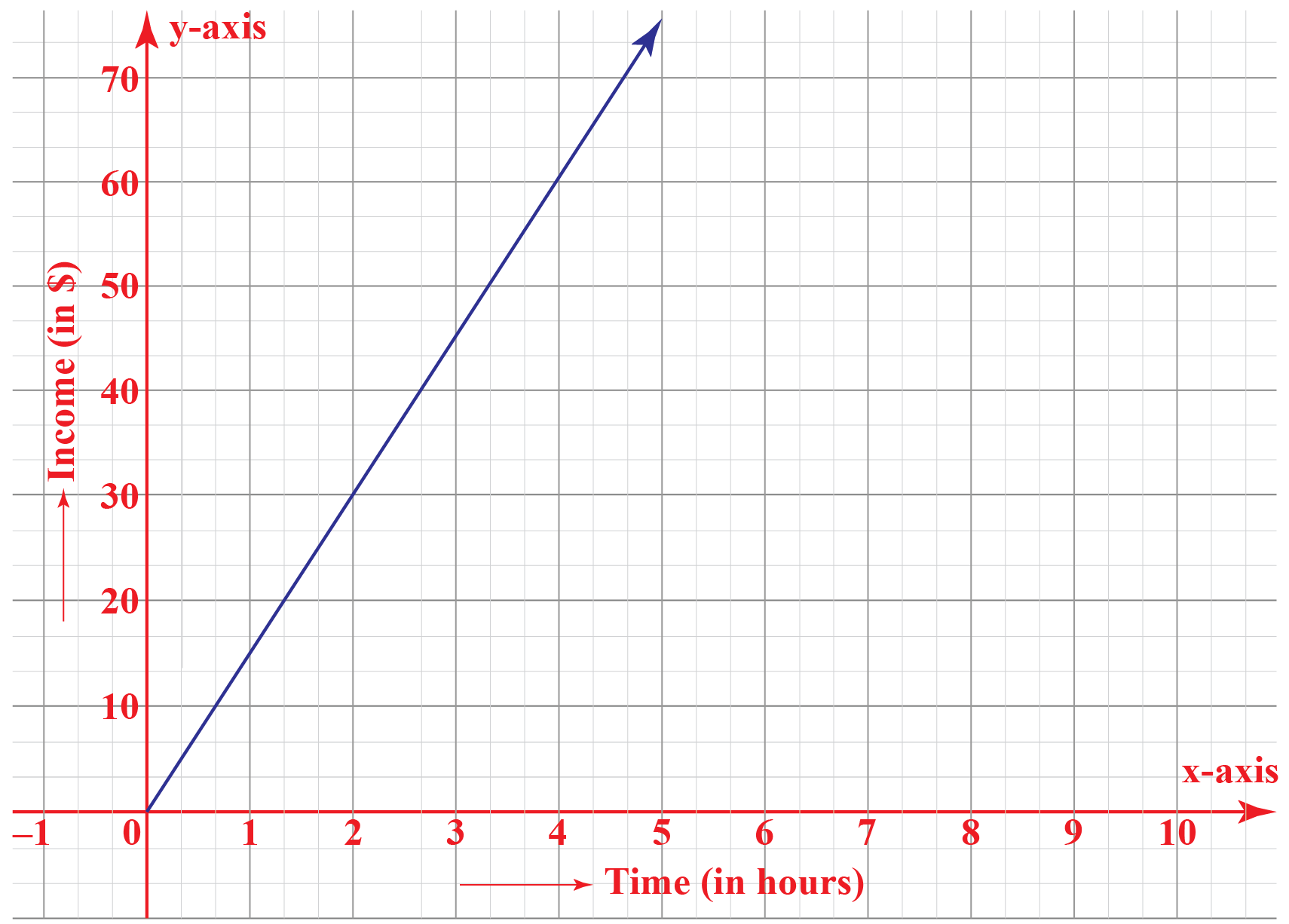
The coefficients α α and β β are typically set to values that will minimize the sum of squared vertical (!) distances between the data points and the corresponding point on the line.
How do you find the linearity of a line. There is not a relationship between the residuals and the \(y\) variable; You only need to check the linearity assumption for continuous predictors. Move your dependent variable to the dependent box.
Visualize your data with a scatterplot. The svd breaks data up into component pieces ranked by magnitude of their contribution to the whole. There are two main types of linear regression:
Place checks in the confidence intervals and descriptives boxes. Linear regression is a regression model that uses a straight line to describe the relationship between variables. It finds the line of best fit through your data by searching for the value of the regression coefficient (s) that minimizes the total error of the model.
Ensure that the estimates and model fit boxes are checked. The linearity assumption can be checked by inspecting the residuals vs fitted plot (1st plot): The presence of a pattern may indicate a problem with some aspect of the linear model.
Linearity is a quantitative assessment of how strongly related a set of data is. How do you check the assumptions of linear regression before modeling? The easiest way to detect if this assumption is met is to create a scatter plot of x vs.
In other words, \(y\) is independent of errors. A linear function, we have seen is a function whose graph lies on a straight line, and which can be described by giving the slope and y intercept of that line. One way to check the linearity is to plot the target versus the predictors for each of the predictors in the dataset.
How to determine if this assumption is met. The rest of the values will be almost zero. Move your independent variable to the independent (s) box.
Finding the least squares line. That is, the red line should be approximately horizontal at zero. We can check the linearity of the data by looking at the residual vs fitted plot.
The ratio of the largest singular number to the second largest. There is a special kind of linear function, which has a wonderful and important property that is. A better unitless option is to use a singular value decomposition (svd).
The input signal x(t) is varied at fixed value of t (let 1 sec). Here is you can check the assumptions of linear regression linearity : $$f(a+b)=f(a)+f(b)$$ and you want to determine whether a particular function $g$ is linear, so you just check whether this property holds.